What Is the FAIR Methodology?
The FAIR methodology is a quantitative risk analysis framework specifically designed for information security and operational risk management. Unlike traditional qualitative approaches, which rely on subjective assessments like "high," "medium," or "low" risk. Think of it as replacing "I feel pretty worried about this" with "There's a 30% chance this could cost us $500,000."
At its core, FAIR evaluates risk through two main factors:
- Loss Event Frequency: Think of this as "how often something bad will happen". (Like how many times a year might we face a security incident?)
- Loss Magnitude: Think of this as "when it happens, what will it cost us". (Both in terms of direct costs like lost revenue and indirect impacts like damage to our reputation)
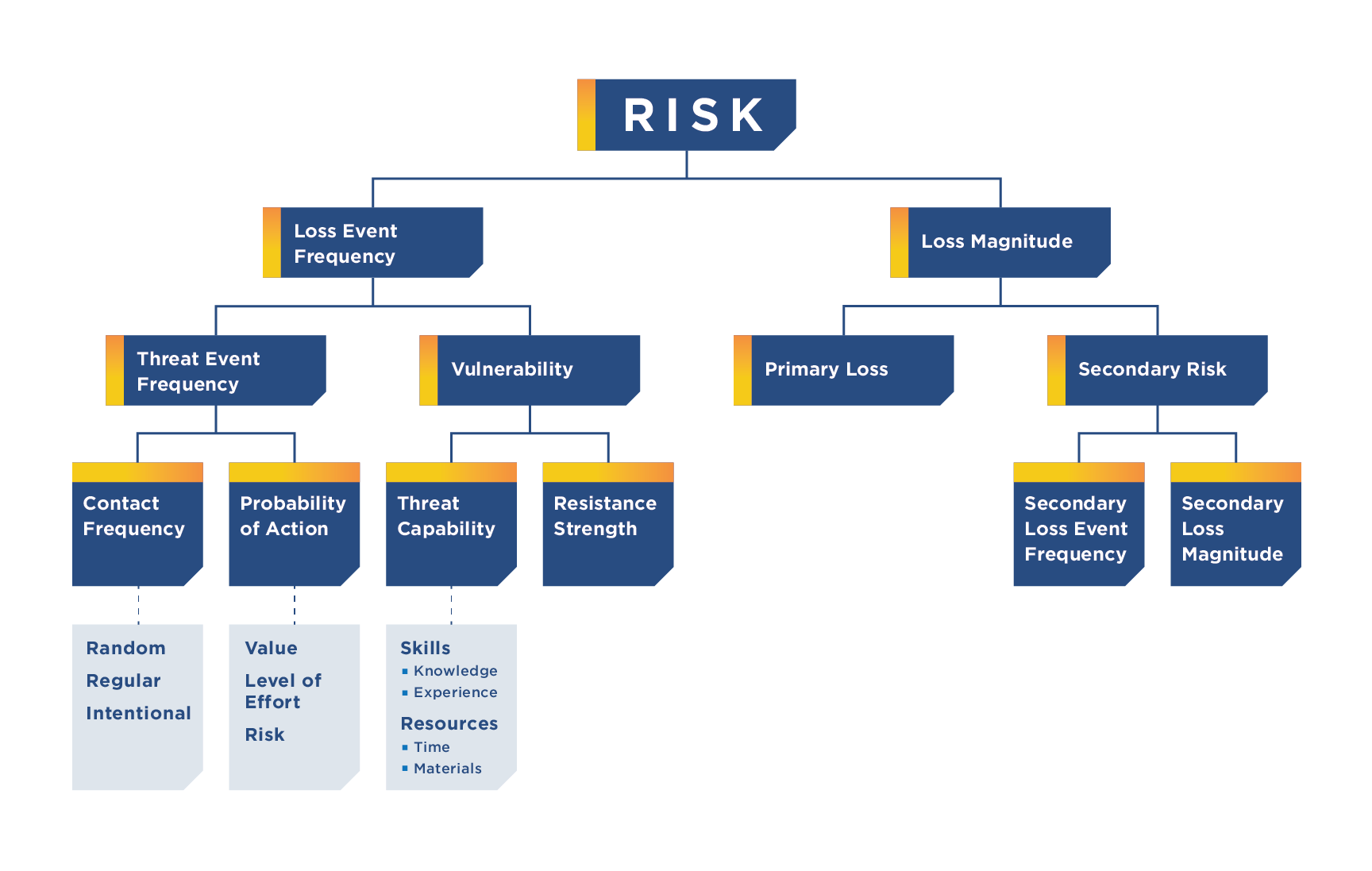
Breaking things down this way helps organizations make smarter decisions about where to invest their security dollars. It's like having a financial advisor for risk management – instead of making decisions based on fear or headlines, you're using real data to guide your choices.
When we add AI to this mix, it's like supercharging the whole process. AI can crunch through massive amounts of data and spot patterns that humans might miss, making these risk calculations even more accurate and easier to scale across an organization.
Benefits of Using AI in FAIR Risk Assessments
- Enhanced Precision and Quantification
FAIR’s strength lies in its quantitative approach to risk assessment, and AI complements this by analyzing large datasets with unparalleled accuracy. AI can identify subtle patterns and correlations in historical data, enabling more precise estimates of both the frequency and magnitude of risk events.
For example, in cybersecurity, an AI system could analyze network traffic, past breaches, and external threat intelligence to predict the likelihood and impact of specific attack vectors. This level of precision would help an organization allocate resources more effectively. - Efficiency and Scalability
Risk assessments conducted manually can be time-consuming and labor-intensive, particularly when large datasets or complex systems are involved. AI automates much of this process, drastically reducing the time required to perform assessments.
For example, an AI-driven FAIR analysis tool can process thousands of cyber threat scenarios in real time, enabling organizations to scale their risk assessment efforts without proportional increases in human resources. This scalability is particularly valuable for enterprises managing global operations or handling diverse risk profiles. - Improved Decision-Making
By combining FAIR’s structured methodology with AI’s predictive capabilities, organizations gain actionable insights to guide decision-making. For instance, AI can use FAIR metrics to prioritize risks based on their financial impact, enabling executives to make informed trade-offs between mitigation costs and potential losses.
This is particularly useful in industries like finance and insurance, where decisions about risk tolerance and resource allocation can have significant financial implications. - Data-Driven Objectivity
Traditional risk assessments often suffer from subjectivity, with outcomes influenced by individual biases or organizational politics. FAIR’s quantitative foundation reduces subjectivity, and AI further enhances objectivity by making data-driven decisions.
For example, an AI system analyzing employee behavior for insider threats can focus solely on quantifiable data—such as unusual access patterns—without being influenced by personal relationships or assumptions. - Continuous Monitoring and Adaptability
One of the key advantages of AI is its ability to operate in real time. When integrated into a FAIR-based framework, AI can continuously monitor risk factors and update assessments as new data becomes available.
For example, an AI system in a FAIR risk assessment model for cybersecurity can adapt to emerging threats, ensuring that the organization’s risk profile remains accurate and up-to-date. - Cost Efficiency
While the initial implementation of AI and FAIR tools may require significant investment, the long-term cost savings can be substantial. Automation reduces the need for manual labor, while more accurate risk assessments minimize unnecessary spending on mitigation strategies.
For example, a FAIR-driven AI system can help an organization avoid overinvesting in low-priority risks or underinvesting in high-impact threats, optimizing budget allocation.
Drawbacks of Using AI in FAIR Risk Assessments
- Data Dependency and Quality Issues
AI systems are only as good as the data they are trained on, and FAIR-based assessments rely heavily on accurate, comprehensive datasets. If the input data is biased, incomplete, or outdated, the results of the assessment can be flawed.
For example, an AI model trained on historical data might fail to account for novel risks or changes in the threat landscape, leading to inaccurate predictions of event frequency or impact. - Complexity and Expertise Requirements
Both AI and FAIR require specialized expertise to implement effectively. FAIR’s quantitative approach can be challenging for organizations unfamiliar with statistical modeling, and AI systems often require data scientists and engineers to develop, maintain, and interpret them.
This complexity can create barriers to entry, particularly for small and medium-sized organizations with limited resources. - Over-Reliance on Automation
While AI enhances efficiency, over-reliance on automated systems can lead to complacency. Human oversight is critical to ensure that AI-driven assessments align with organizational priorities and ethical considerations.
For instance, an AI system might flag a low-probability, high-impact risk as a priority, but human judgment may be needed to contextualize its significance within the broader organizational strategy. - Transparency and Interpretability Challenges
Many AI models, particularly those based on machine learning, operate as “black boxes,” making it difficult to understand how they arrive at specific conclusions. This lack of transparency can undermine trust in the risk assessment process and complicate compliance with regulations that require explainability.
For example, if a FAIR-based AI system predicts a high likelihood of a specific cyberattack, stakeholders may demand a clear explanation of the factors contributing to this assessment. - High Implementation Costs
While AI can reduce long-term costs, the upfront investment in developing and deploying AI-driven FAIR tools can be prohibitive. Organizations must consider the costs of data collection, model training, software development, and ongoing maintenance.
Smaller organizations may struggle to justify these costs, particularly if they lack the scale to fully leverage AI’s capabilities. - Ethical and Privacy Concerns
AI-driven risk assessments often involve the collection and analysis of sensitive data, raising ethical and privacy concerns. FAIR’s focus on quantitative metrics may require detailed data about individuals or systems, increasing the risk of misuse or unauthorized access.
For example, in a FAIR-based AI system assessing insider threats, employee monitoring could inadvertently infringe on privacy rights, leading to ethical dilemmas and potential legal challenges.
Balancing the Benefits and Drawbacks
When implementing FAIR risk assessments using Artificial Intelligence in SimpleRisk, we were looking for ways to maximize the benefits while eliminating the pain points typically associated with both AI and FAIR risk assessments. We began by optionally allowing the user to answer a number of questions that help to guide the context that the AI uses to perform its assessments. A data breach has very different ramifications for a startup than for a publicly-traded international corporation. We then combined the contextual insight from the user with one of the largest AI data models on the planet, to provide a comprehensive set of expected outcomes. Users are provided with a transparent description of what decisions were made for the risk assessment and why. This process allows the AI to take a qualitative analysis performed in SimpleRisk and quickly obtain a contextualized quantitative assessment that removes all of the subjectivity that typically wreaks havoc on quantitative models, and does it in a way that is far more scalable and cost-effective than traditional FAIR assessments.
Conclusion
Using AI to perform risk assessments under the FAIR methodology offers numerous advantages, including enhanced precision, efficiency, and objectivity. By combining FAIR’s structured framework with AI’s data-driven capabilities, organizations can gain deeper insights into their risk profiles and make more informed decisions.
However, these benefits come with challenges such as data dependency, complexity, and ethical concerns. By addressing these drawbacks through careful planning and oversight, organizations can harness the full potential of AI-driven FAIR risk assessments, ensuring that they are not only effective but also fair, transparent, and responsible.